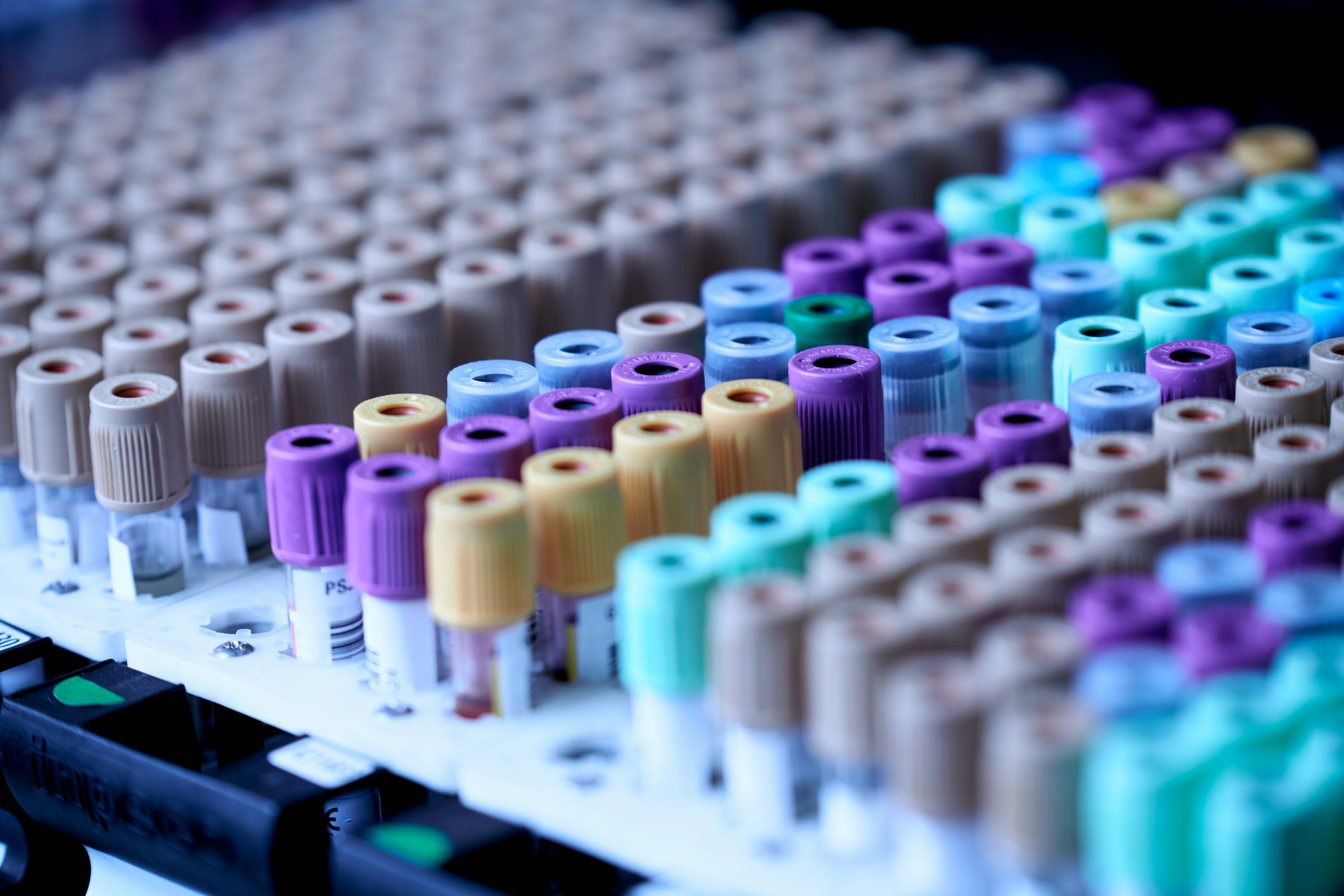
Machine Learning in Healthcare: Shaping the Future of Medicine
The healthcare industry is experiencing a paradigm shift driven by the transformative power of artificial intelligence (AI). At the forefront of this revolution stands machine learning (ML), a technology redefining patient care, drug discovery, and medical research.
The Evolving Landscape of AI in Medicine: From Expert Systems to Deep Learning
The integration of artificial intelligence (AI) in the medical field has been a fascinating journey marked by continuous learning and adaptation. In the early days, the 1970s and 80s, AI's role primarily involved expert systems and rule-based programs designed to mimic the decision-making of medical professionals. These systems, like MYCIN for bacterial infection diagnosis, laid the groundwork for future advancements in machine learning algorithms.
The 1990s saw the rise of statistical learning, where AI algorithms analyzed vast amounts of medical data to identify patterns and make predictions. This era brought forth applications like early disease detection through medical imaging analysis.
The 21st century ushered in the age of deep learning, where AI models inspired by the human brain's structure achieved remarkable breakthroughs. Deep learning has revolutionized medical image analysis, enabling earlier and more accurate cancer detection, as well as personalized medicine through the analysis of genetic and patient-specific data.
Today, AI in healthcare continues to evolve, venturing into new frontiers like robotic surgery with AI assistance, remote patient monitoring with wearable devices and sensors, and even mental health diagnosis and treatment support.
By analyzing vast amounts of healthcare data, from electronic health records (EHRs) to medical images and patient records, ML algorithms learn to identify patterns, predict outcomes, and personalize treatment plans.
12 Examples of Machine Learning in the Healthcare Industry
1. Early Disease Detection: One of the most promising applications of AI is in the early detection of diseases. Deep learning models and algorithms are trained on vast amounts of medical imaging data, like mammograms and X-rays, to identify subtle abnormalities that might indicate early-stage cancer. This allows for earlier intervention and treatment, significantly improving patient outcomes.
- Deep learning algorithms analyze medical images like mammograms and X-rays to detect cancer at its earliest stages, significantly improving patient prognosis.
2. Personalized Medicine: AI is transforming healthcare from a one-size-fits-all approach to personalized medicine. By analyzing a patient's medical history, genetics, and lifestyle, ML models can predict treatment responses and tailor therapy plans for optimal efficacy and reduced side effects. This personalized approach holds immense potential for improving patient outcomes and quality of life.
- ML models analyze patient-specific data, including genetics, medical history, and lifestyle, to predict treatment responses and tailor care plans for optimal outcomes.
3. Streamlined Clinical Trials: Drug discovery and development are often lengthy and expensive processes. AI is accelerating this process by analyzing clinical data much faster and more effectively. ML algorithms can identify promising drug candidates, predict side effects, and optimize trial design, leading to faster development and reduced costs for bringing new medications to market.
- ML algorithms analyze clinical trial data faster and more effectively, accelerating drug discovery and development while reducing costs.
4. Predictive Analytics for Risk Assessment: AI can help predict a patient's risk of developing chronic diseases like diabetes or heart failure. By analyzing factors like blood pressure, cholesterol levels, and family history, ML models can identify individuals at high risk, allowing for proactive interventions and preventive care strategies. This proactive approach can prevent disease onset and improve overall health outcomes.
- ML models identify patients at high risk for chronic diseases like diabetes or heart failure, allowing for proactive interventions and preventive care.
5. Automated Medical Imaging Analysis: Radiologists are often faced with a large volume of medical images to analyze, leading to potential fatigue and missed diagnoses. AI-powered tools are assisting radiologists by automating tasks like tumor segmentation and anomaly detection. This frees up valuable time for radiologists to focus on complex diagnoses and improve overall workflow efficiency in clinical practice.
- ML algorithms automate tasks like tumor segmentation and anomaly detection in medical images, freeing up radiologists' time for complex diagnoses.
Beyond the examples mentioned, here are a few more ways ML is impacting healthcare:
6. Virtual Assistants for Patient Support:
- Natural language processing (NLP)-powered chatbots provide patients with 24/7 support, answer questions, and offer medication reminders, improving patient engagement.
7. Administrative Efficiency & Fraud Detection:
- ML algorithms streamline administrative tasks like insurance claims processing and identifying fraudulent activities, reducing costs and improving healthcare system efficiency.
8. Robotic Surgery with AI Assistance:
- Robotic surgery systems powered by ML algorithms allow for minimally invasive procedures with increased precision and reduced risks, leading to faster recovery times.
9. Remote Patient Monitoring:
- Wearable devices and sensors collect real-time patient data, while ML algorithms analyze it to detect early signs of health deterioration and improve remote care delivery.
10. Drug Discovery & Development:
- ML accelerates the drug discovery process by identifying promising drug candidates, predicting side effects, and optimizing clinical trials.
11. Mental Health Diagnosis & Treatment:
- ML algorithms analyze speech patterns and facial expressions to detect depression and anxiety, aiding in early diagnosis and personalized treatment plans.
12. Public Health & Epidemic Management:
- ML models analyze data from various sources to track disease outbreaks, predict future trends, and inform public health interventions.
These are just a glimpse of the immense potential of machine learning applications in healthcare. As this technology continues to evolve, we can expect even more transformative applications that will revolutionize patient care, empower healthcare professionals, and ultimately lead to a healthier future for all.
Key Advantages of Machine Learning in the Healthcare Industry
- Improved patient outcomes: Early detection, personalized medicine, and better risk assessment lead to improved patient survival rates and overall well-being.
- Enhanced clinical decision support: ML algorithms provide healthcare providers with data-driven insights to support informed clinical decisions.
- Reduced healthcare costs: Streamlined processes, optimized resource allocation, and fraud detection contribute to significant cost savings in the healthcare system.
- Accelerated medical research: ML unlocks faster and more efficient drug discovery, clinical trials, and personalized treatment development.
However, challenges remain in the widespread adoption of machine learning systems in healthcare. Data privacy and security concerns, the need for robust data infrastructure, and ensuring ethical implementation are crucial considerations.
As we move forward, responsible development, collaboration between data scientists and healthcare professionals, and ongoing education are vital to unlocking the full potential of machine learning for a healthier world.